With absolute clarity, data scientist is currently the highest paying job role in the market. But within Pakistan, the early professionals entering in the market doesn’t seem to land on the expected paid job they once dreamed. The reason for this ironic turn lies in the kind of career approach rookies adopt when setting off in their data science journey.
This article addresses the right career approach of an individual seeking guidance in the data analytics field. Our mentor for today’s career guidance is Mr. Ali Raza Anjum who is a shrewd data scientist serving Pakistan’s telecom industry for over 10 years. Ali Raza Anjum is also co-founder and managing director of a training and consultancy platform Dice Analytics.
Without further ado let’s dive into a decade’s-long experience advice from Mr. Ali.
Skills required to become a Data Scientist
When it comes to guiding the skillset of a data scientist, Dice Analytics presents its ultimate work flow chart that describes three steps of a data scientist job role. Each step identifies a skills area which is required by the data scientist.
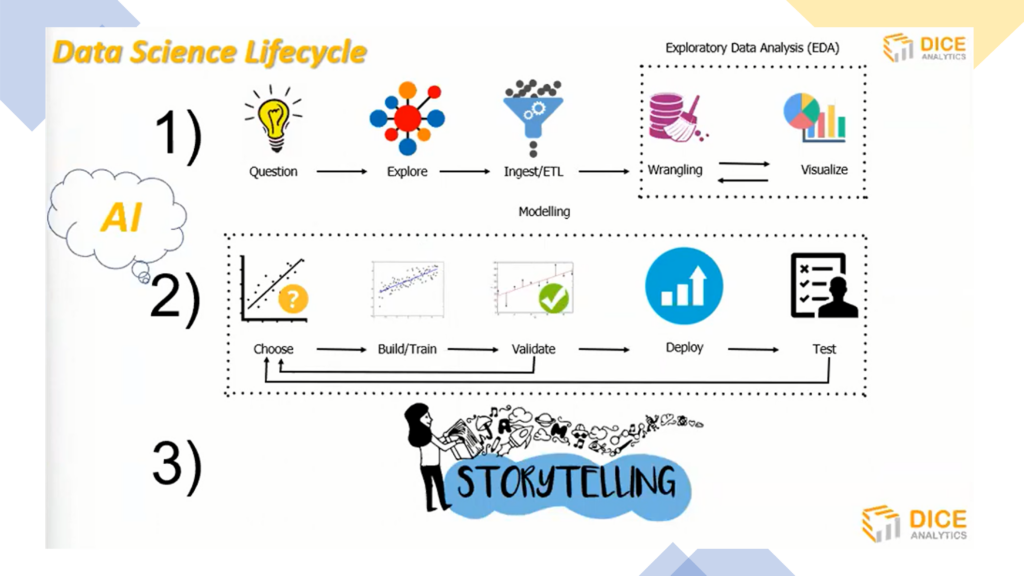
At this point, you will recognize that industrial experts are looking for data scientists who have the skillset of:
1-) Data Engineer who fetches and cleanses the relevant data from a database
2-) Machine learning expert who builds and runs ML models
3-) Storyteller who demystifies technical knowledge for non-technical staff
Missing any one of these, you can’t land on a good data science job.
The first step of Data Engineering is often skipped by Pakistani individuals where they directly jump to the second step of the machine learning area.
At this point we want to break a common myth among youth of Pakistan that is “if you learn Python then you become a data scientist“. However, the truth is that learning Python (which is a de facto machine learning language) only enables you to have over 30% data science knowledge. The Remaining 70% is still missing. So, let’s sequentially walk through the skills you require to become a data scientist which industrial experts are looking for.
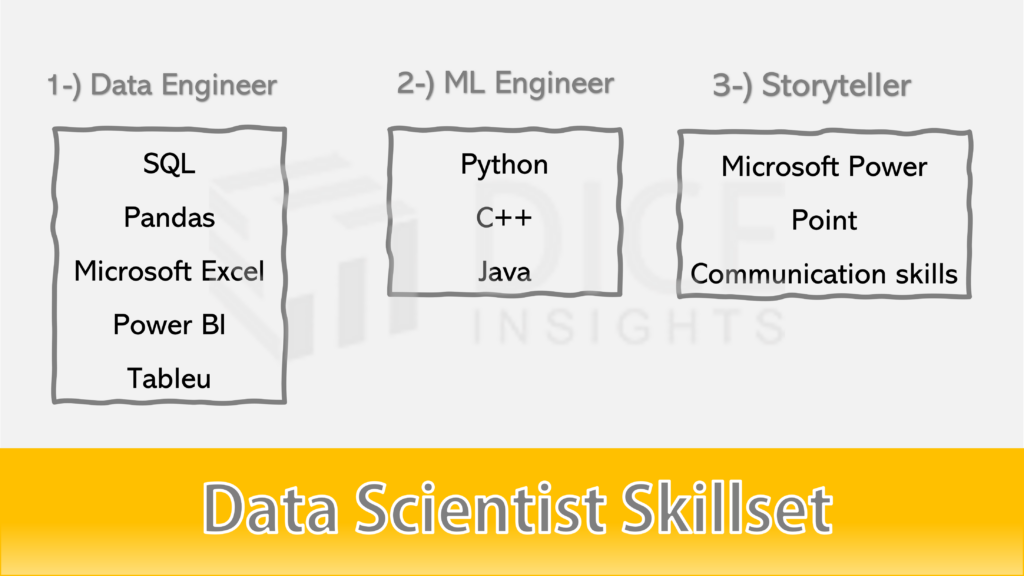
Step 1: Data Engineering Skills
Data engineering is the very start of the data science workflow. It’s defined as the ability to extract, clean, and store the right data to answer a specific set business problems. Formally, the operation is known as ETL (extract, transform and load of the data) or in some cases ELT (extract, load, and transform). In case of a data warehouse- DW, the data engineering work is not limited to ETL tasks, and involves the dimensional modeling in the presentation area of the DW architecture.
At first, all the most relevant data is fetched from the operational systems of the organization. These systems are the OLTP (Online Transaction Processing) systems and support transactions of data such as insert, and update queries. This raw data from OLTP sources is stored in a single repository that could be a database or for the case of reaching towards analytics goal, a data warehouse.
This huge body of raw data before the ETL process might carry anomalies such as redundancies, making it unsuitable for efficient transnational query processing. So, a data engineer will transform and organize this data into smaller and more manageable data tables to support simple and fast query processing. These hundreds of small tables are easy to understand, and could be updated without hurting data integrity.
Finally the data could be loaded and further cleaned in the data wrangling stage. An example is the merging of the previously normalized data sets to enable data visualization.
The organized form of data enables faster query performance in the database and makes it easy for data scientists to load error-free data in their machine learning algorithm.
Skill Set: SQL to query data from a database. Python led Pandas, Microsoft Excel, Power Query Editor (an ETL tool from MS Power BI), and Tableau for data cleaning. All these tools could be used for data visualization as part of data engineering.
Step 2: Machine Learning Skills
After cleansing and visualizing the data, this data is ready for any ML operation.
While AI is a huge area that enables machines to mimic human behavior, ML is a subset of AI and deals with enabling machines to learn. A computer can be made to learn anything! That’s how powerful ML is. A data scientist at this phase just collects relevant data (that’s already engineered to clean) and applies statistical modeling to find hidden trends. These trends are the information that is critical to your application and enables decision making.
You must acquaint yourself with the many ML methods available in theory. These include, supervised and unsupervised learning, semi supervised learning, and reinforcement learning.
The first stage in ML engineering is to choose a statistical model that is based on a mathematical theory. A model studies the structure of your data which is then used as the pattern. The model can be modified as per the output accuracy in following the second and third step of ML workflow.
Second, the chosen model is trained with already labeled data (in supervised learning). Labeled data is the known data and acts as training material for the algorithm. In unsupervised learning, there’s no labeled data and the algorithm finds out itself.
Third, a validation test is performed on the model and compared with the known data to evaluate accuracy. If the system doesn’t allow correct recognition, then the model is modified until a robust pattern is found.
After validation, in the fourth step, an ML engineer deploys the system for its actual use in the application.
Fifth step again shows the iterative approach of ML to learn. Using a feedback loop, the learning can be easily automated.
Skill Set: Python and C++ used in ML tools: Pytorch and TensorFlow. Java used in ML tool KNIME.
Step 3: Storytelling Skills
Finally, after a lot of discerning work with data, a data scientist now has to shift from a scientist to a storyteller. For this, the data scientist needs to come out from the technical jargon and actually think of a business strategist who is unaware of the technicalities of data analysis.
At this phase, a data scientist finds answers to the questions: What would a non-technical person understand from the insights? What does an insight mean for the business? How could these insights be transformed into actionable strategies that could benefit the business? And so on.
Skill Set: Microsoft Powerpoint, sound knowledge, ability to understand things clearly, and knowing the art of public speaking.
Example: Job Role of a Data Scientist
What you see in the image below is a famous character Ravan, who has ten heads each representing a separate role. This famous metaphor is used for the job role of a data scientist.
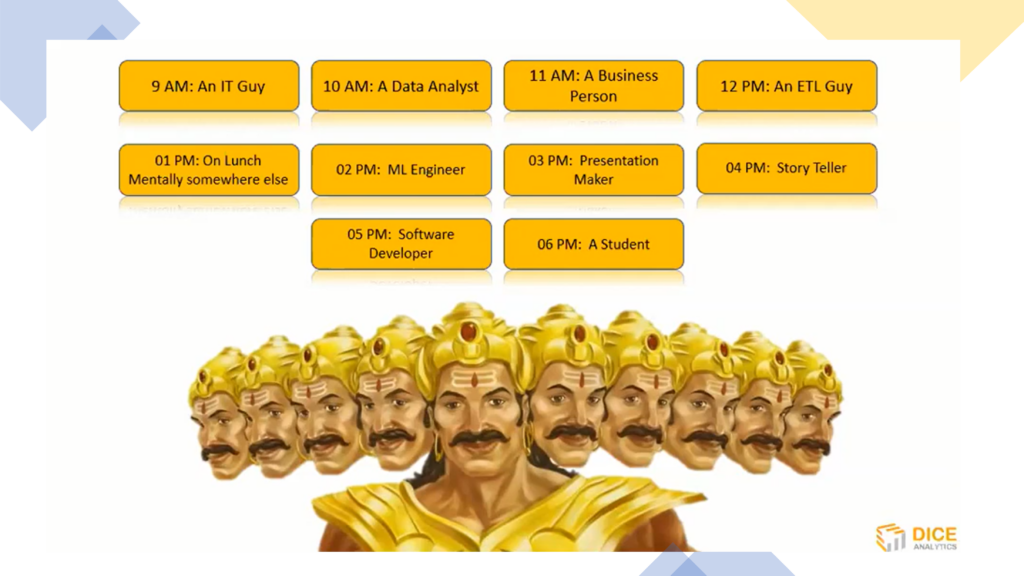
At the start of job hours, a data scientist is an IT guy who evaluates the working of their data model which was built a day before. Once the model is evaluated, finding insights as a data analyst is the next role of the data scientist. Successfully finding the analytic trends from the data the data scientist will be given a new task which takes us to the next role of Business person.
As a business person, a data scientist is encountered with diverse business questions. Based on the new business problem, the data scientist will now perform the role of a data engineer, an ETL guy. Following this, the data engineer will now shift their role to an ML engineer who will select a machine learning algorithm and feed data in it to extract insights.
After the insights extraction, the data scientists prepare for the presentation and adopt the role of a story teller. Once approved by the business executives, the data scientists advance to deploy the ML model that was designed earlier.
Lastly, as the day ends, the data scientist is a student who continues to explore more possibilities of technology advancements. Keeping updated with the latest data science news helps a data scientist to dig out new ways to clean, and train data for data analysis operations, and finding out provocative ways to deliver to the business management.
To conclude at this note, learning data science is an everlearning job. The learning curve of a data scientist grows with time meaning that new skills shall be learnt regularly and more quickly than before.
Wish you good learning!
We hope that this career guide has offered some valuable information on building a data science career in Pakistan and that it will help you in taking the right step in your data science journey. The career guide is also available in our latest video presentation by Mr. Ali.
Wish you good learning!
About Ali Raza Anjum
Ali Raza Anjum is co-founder and managing director of Pakistan’s leading training and consultancy platform- Dice Analytics. Mr. Ali has extensive experience of working with all the Top Telecom Companies of Pakistan. Under Dice Analytics, the discerning career advice from Mr. Ali has led hundreds of rookies to land on the right job roles in their data analytics career. He has the privilege of mentoring & guiding non-Coders nationwide so that they can effortlessly transition in the field of Data Science and build a strong career.
Mr. Ali Raza Anjum can be approached via LinkedIn.